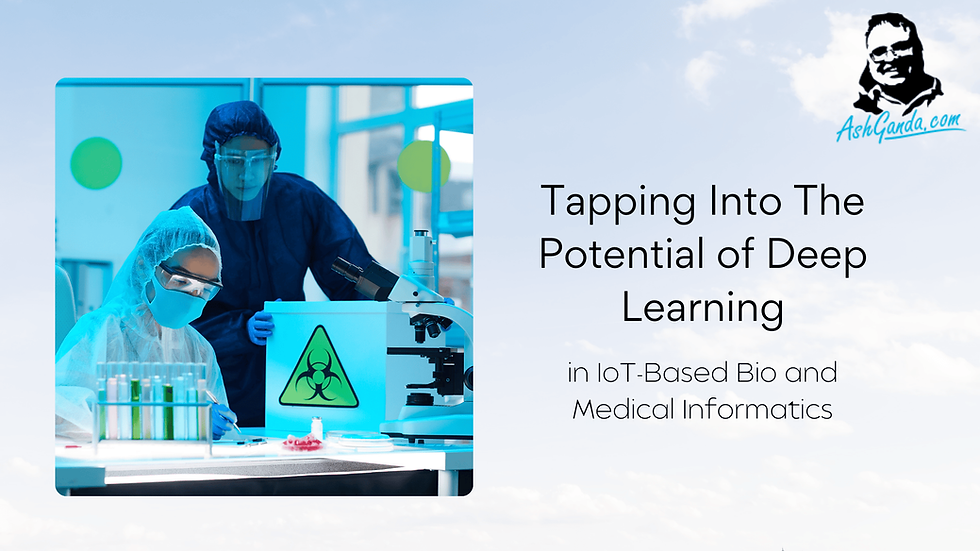
In recent years, the integration of deep learning (DL) with the Internet of Things (IoT) has taken the bio and medical informatics field by storm. This synergy has enabled unprecedented advancements in healthcare by leveraging real-time data analysis and predictive capabilities. This article delves into the applications, challenges, and future prospects of DL in IoT-based bioand medical informatics.
The Intersection of Deep Learning and IoT in Healthcare
The convergence of DL and IoT technologies offers great potential for bioinformatics and medical informatics. DL algorithms are great at handling large amounts of complex data, making them perfect for analyzing continuous information from IoT devices. This integration facilitates various applications, including:
Diagnosis and Treatment Recommendations: DL models can analyze patient data from wearable devices to provide real-time diagnosis and personalized treatment plans.
Clinical Decision Support: By processing large datasets from electronic health records (EHRs) and other sources, DL systems can assist healthcare professionals in making informed decisions.
Image Analysis: DL techniques, particularly convolutional neural networks (CNNs), are highly effective in interpreting medical images, aiding in the detection of diseases such as cancer.
Wearable Monitoring: IoT devices equipped with DL algorithms can monitor patients' vital signs continuously, alerting healthcare providers to any anomalies.
Drug Discovery: DL models can analyze biological data to identify potential drug candidates, accelerating the drug development process.
Challenges in Implementing Deep Learning in Bioinformatics
Despite its potential, integrating DL with IoT in bioinformatics presents several challenges:
Data Privacy and Security: The sensitive nature of medical data necessitates robust security measures to protect against breaches.
Data Quality and Availability: High-quality labeled datasets are essential for training accurate DL models, yet they are often scarce in the medical field.
Interpretability: DL models are often seen as "black boxes," making it difficult to understand how they arrive at specific conclusions. This lack of transparency is a significant hurdle in medical applications where trust and accountability are paramount.
Computational Resources: Training DL models requires substantial computational power, which can be a barrier in resource-constrained environments.
Future Directions and Innovations
To overcome these challenges, researchers are exploring several innovative approaches:
Federated Learning: This technique allows models to be trained across multiple decentralized devices without sharing raw data, thus enhancing privacy.
Explainable AI (XAI): Developing methods to interpret DL models' decisions can increase trust and facilitate their adoption in clinical settings.
Transfer Learning: By leveraging pre-trained models on large datasets, transfer learning can improve performance on tasks with limited data availability.
Blockchain Technology: Integrating blockchain with IoT systems can enhance data security by providing a tamper-proof ledger for medical records.
Conclusion: Potential of Deep Learning in Bio and Medical Informatics
The integration of deep learning with IoT has the potential to revolutionize bioand medical informatics by enabling more accurate diagnoses, personalized treatments, and efficient drug discovery processes. However, addressing challenges related to data privacy, interpretability, and computational demands is crucial for realizing this potential. As research continues to advance these technologies, the future of healthcare looks increasingly promising with more intelligent and connected systems.
Note: This post is based on the journal article by Amiri et al. (2024).
References
Amiri, Z., Heidari, A., Navimipour, N. J., Esmaeilpour, M., & Yazdani, Y. (2024). The deep learning applications in IoT-based bioand medical informatics: A systematic literature review. Neural Computing and Applications, 36(5757–5797). https://doi.org/10.1007/s00521-023-09366-3
Comments